Databricks and Snowflake overlap in many areas. Firms deploying both need to clearly demarcate the epics and use case journeys to be supported by the technologies. The last thing you want is to create 2 data lakes for example, or construct 2 ecosystems which are doing similar activities.
Databricks: Analytics
Databricks is a unified analytics platform, encompassing a comprehensive range of features for data engineering, data science, and machine learning. Its foundation lies in Apache Spark, a distributed computing framework designed for large-scale data processing. Databricks seamlessly integrates Spark with Delta Lake, a data storage format that ensures data reliability and integrity.
Snowflake: Cloud datawarehouse
Snowflake, on the other hand, excels as a cloud-native data warehouse, and is a SaaS, sitting on top of AWS or Azure infrastructure, and delivering a secure and scalable platform for data warehousing and business intelligence. Its architecture separates storage and compute, enabling independent scaling of each resource to meet fluctuating data volumes and processing demands. This architecture also eliminates the need for infrastructure management, simplifying operations and reducing costs.
Key Differentiators: A Comparative Analysis
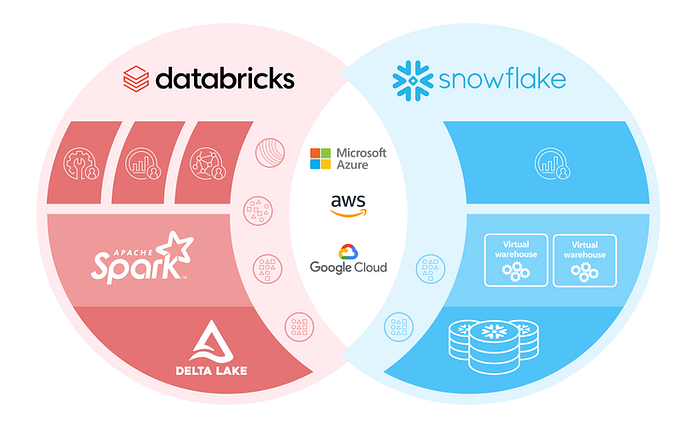
Some comparison notes between Databricks and Snowflake below.
Data Processing:
- Databricks: Databricks excels in data processing, particularly for real-time data streaming and machine learning applications. Its Apache Spark foundation provides powerful data processing capabilities, enabling complex data transformations and analyses.
- Snowflake: Snowflake focuses on data warehousing, providing a robust platform for storing and analyzing structured data. Its SQL-based interface caters to business intelligence users, enabling them to easily query and visualize data.
Data Ingestion:
- Databricks: Databricks supports a wide range of data ingestion methods, including streaming data sources, cloud storage platforms, and on-premises databases. Its Delta Lake format facilitates efficient data ingestion and processing.
- Snowflake: Snowflake offers seamless data ingestion from various sources, including cloud storage, databases, and semi-structured data files. Its architecture ensures data integrity and reliability during ingestion.
Ease of Use:
- Databricks: Databricks’ interface is geared towards data engineers and data scientists, providing a collaborative environment for data exploration and experimentation. However, its complexity may pose challenges for less technical users.
- Snowflake: Snowflake’s user-friendly interface simplifies data warehousing tasks, making it accessible to business users and analysts. Its SQL-based approach is familiar to those with traditional data warehousing experience.
Pricing:
- Databricks: Databricks employs a usage-based pricing model, where costs are determined by the amount of data stored, compute resources utilized, and features used.
- Snowflake: Snowflake also utilizes a usage-based pricing structure, but its pricing model is more complex, considering factors such as storage, compute, data transfer, and queries.
Choosing the Right Platform: A Decision Framework
The choice between Databricks and Snowflake ultimately depends on the specific needs and technical expertise of the organization. Here’s a decision framework to guide your choice:
- Data Processing Requirements: If your organization prioritizes real-time data processing, machine learning, and data engineering, Databricks is a strong choice.
- Data Warehousing Needs: If data warehousing, business intelligence, and structured data analysis are your primary focus, Snowflake is well-suited.
- Technical Expertise: If your team comprises data engineers and data scientists with strong technical skills, Databricks’ flexibility can be advantageous.
- Ease of Use: For teams with less technical expertise or a preference for SQL-based interfaces, Snowflake’s user-friendliness is a valuable asset.