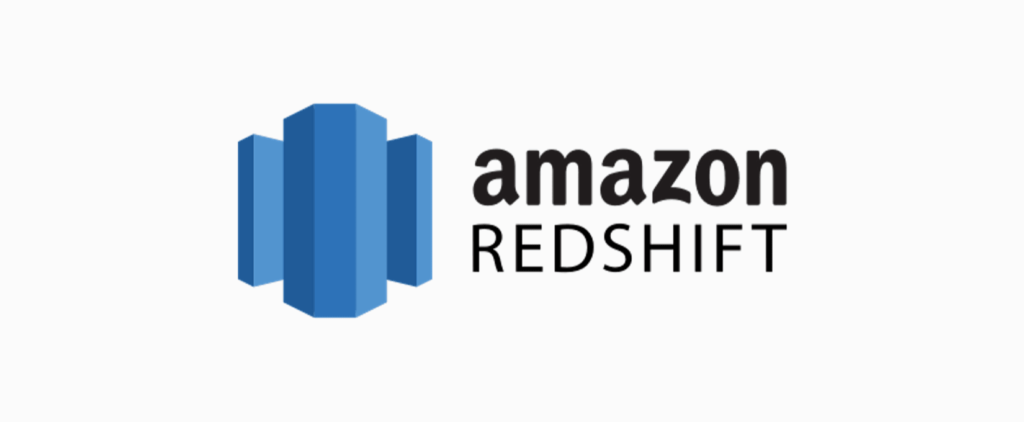
Amazon Redshift
Redshift is a fully managed data warehousing service designed for online analytical processing (OLAP) workloads. It is optimized for high-performance analysis and querying of large datasets. Redshift has clusters, and stores data in a columnar format and uses a massively parallel processing (MPP) architecture.
Data Storage
Redshift divides data across the cluster in multiple nodes and slices. Each node in the cluster contains a subset of the data. The data is distributed using a key distribution style or an even distribution style, based on the defined distribution key. Within each node, data is stored in columns rather than rows, allowing for efficient compression and query performance.
Key Differences
Columnar Storage
Redshift stores data column-wise, enabling faster query execution for analytical workloads compared to traditional row-based databases.
MPP Architecture
Redshift employs a massively parallel processing architecture to distribute query execution across multiple nodes, allowing for high concurrency and performance.
Schema-on-Read
Redshift follows a schema-on-read approach, which means that the data does not need to be fully structured upfront, allowing for flexibility in querying semi-structured and structured data.
Use Cases
Redshift is ideal for data warehousing and analytics scenarios, such as business intelligence reporting, ad hoc analysis, and complex analytical queries on large datasets.
Enterprise Use Case Example
A retail company can use Redshift to analyze vast amounts of sales data to:
- Identify customer purchasing patterns,
- Optimize inventory management,
- Make data-driven business decisions
Quick connection example (Python):
import psycopg2
# Connect to Redshift cluster
conn = psycopg2.connect(
host='your-redshift-endpoint',
port=5439,
dbname='your-database-name',
user='your-username',
password='your-password'
)
# Create a cursor
cursor = conn.cursor()
# Execute a query
cursor.execute('SELECT * FROM your_table')
# Fetch the results
results = cursor.fetchall()
# Close the cursor and connection
cursor.close()
conn.close()
Data Compression
Redshift supports various compression algorithms, including LZO, Zstandard, and Run-Length Encoding (RLE). Compression reduces storage space and improves query performance by minimizing I/O operations.
Performance and Retrieval Time
Redshift’s MPP architecture and columnar storage provide high-performance query execution. By distributing data across multiple nodes and using columnar storage, Redshift minimizes data retrieval time for analytical queries.
Concurrency Scaling
Redshift offers automatic concurrency scaling, which dynamically adds and removes compute capacity based on query load. This ensures that multiple concurrent queries can be executed efficiently without resource contention.
Workload Management
Redshift provides auto workload management (WLM) to manage query queues and control resource allocation. WLM enables setting different query priorities, allocating memory, and defining query execution time limits.
Misconfiguration Pitfalls and Best Practices
Data Distribution and Sort Keys
Choosing an appropriate distribution and sort key is crucial for query performance. Improper distribution can result in data skew and slow down queries.
Vacuuming and Analyzing
Regularly running the VACUUM and ANALYZE commands is essential to maintaining optimal query performance and statistics.
Compression Encoding
Selecting the appropriate compression encoding based on data characteristics can significantly reduce storage costs and improve performance.
Monitoring and Optimization
Monitoring query performance, analyzing execution plans, and optimizing queries based on Redshift’s best practices help ensure efficient data retrieval.
For more detailed information, you can refer to Amazon’s documentation: Amazon Redshift Documentation.